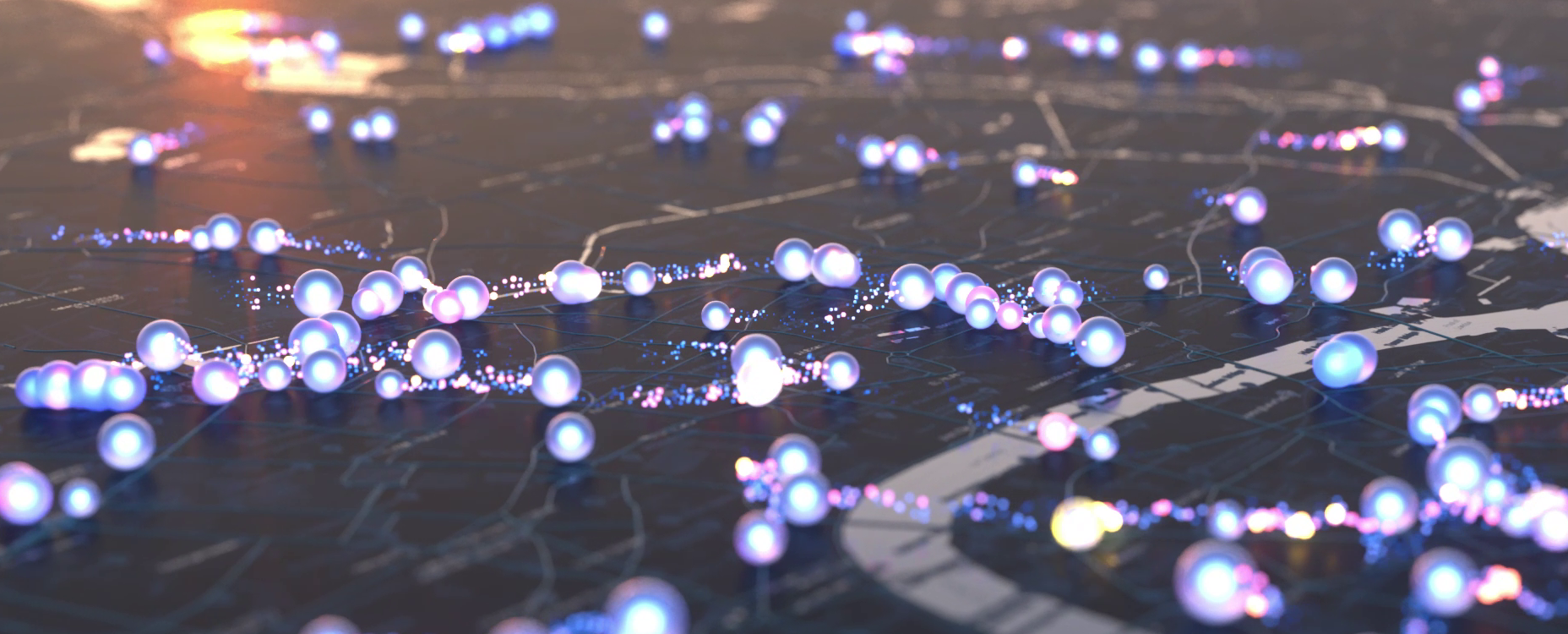
#TransitVizThursdays | Innovative Transit Dataviz
Earlier this year I started posting on a fairly regular basis about some of the work I do at Ito World, mostly revolving around transit dataviz in one way or another. The idea was to post on Thursdays hence the #TransitVizThursdays. The idea was born so I could keep up the cadence of creating animated dataviz; exploring new techniques, reviving old projects and keeping up to date with any new transit-orientated datasets to be released into the wild. I also wanted to spark conversation about techniques and datasets too.
I guess the initial idea of a weekly post was soon replaced by a more flexible approach to a couple a month but the idea stayed the same. The below videos are some of the animations I have created over the past year with a little bit of an explainer for each one. I'll keep adding them as I produce them.
#TransitVizThursday: ⚡ London's EV Buses ⚡
I started looking into the possibility of identifying EV vehicles in BODs over the weekend and (with help from Robin Hawkes previous experiments in EV) found a nice audit from TFL which identified electric vehicle IDs. It didn't take long to throw this 'experimental' viz together showing bus movement for about an hour on Monday morning.
⚗ The size of the sphere is random for now just to create some variance but it could be linked to any number of metrics; on time performance, occupancy, speed etc.
📊 It would be interesting to take this to a more analytical level and start looking at the proportions of ev to normal vehicles or saving on emissions etc.
#TransitVizThursday: LA Speed Analytics
⚗ I experimented with visualizing bus speed patterns in LA this week using two alternative designs. Speed is a straightforward metric that provides some insight into congestion and road conditions.
📊 The first visual uses a lollipop style (for lack of a better term) to show the fluctuation of speed for a rolling hour time caliper. Higher lollipops represent faster bus speeds, and vice versa. I added light emission to highlight lower speeds, with bright yellow indicating slower buses. The graph widget displays the rolling average speed for the entire region.
🗺 The second visual is a bit more experimental and uses a dynamic net heatmap to highlight where low-speed & high-density buses are. The time caliper is upped to 3 hours with a date range of 1 week. Each metric was given a 50% weighting so the peaks show where slow buses are but also where they are bunched together. Again the graph widget shows the rolling average speed for the region.
💡 Speed is a tricky metric to utilise solely as a proxy for road condition, low speeds could be congregated around bus hubs/stops (visible as white spikes on the map). Ideally, this visual needs to include historic road speeds to validate the 'why' to some of the patterns of speed.
⚡ These visuals are all experimental and have pot-holes to them but provide a nice spatial playground for experimental animations. If you want to learn more about the 'how' and 'why' behind these visuals check out the below link for my upcoming presentation at On Data And Design: https://lnkd.in/ejJ5nWN4
I've been looking for an excuse to use GHSL data for a while now (https://lnkd.in/eZUcj9et) - #WorldPopulationDay seemed like a good opportunity!
#TransitVizThursday: The Beauty of the Bus | Milan (2023 edition)
⚗ Slightly different take on #transitvizthursday today based on a personal project I'm iterating on. A few years ago I made a visualisation of GTFS models for various cities, abstracted in a way that played around with the tension of the routes. I wanted to create a network of elegant curves and have the scheduled services follow those curves to create a kind of mesmerising animation.
⚡ This version takes that and elevates the lines - creating a complex, almost organic, depiction of these buses as they begin and end their journeys around Milan.
📊 I've shared a few insightful visuals but this one firmly sits in the #DataArt camp and is more an experimentation in abstract visuals.
I have a few cities in mind but if anyone has any ideas on interesting transit systems let me know!
#TransitVizThursday 🗺 Expanding upon my previous post about Census 21 travel-to-work patterns, this more comprehensive version spans across much more of the UK. The flow data is aggregated to MSOA level, with higher arcs conveying larger flows, and particle movements revealing travel directions.
I also experimented with two different colour treatments covering different parts of the UK. I find flow data fascinating and it is always a challenge to visualise in a clear way but 3d mapping techniques coupled with animation bring these datasets to life.
#TransitVizThursday Brighton Occupancy DataViz
🚌 Summer holidays are slowing the cadence of my weekly transit #dataviz posts but this one from the locker is a nice example of how innovation in visual design can help reveal patterns.
🚏 The visual uses clustering and rigid body dynamics to showcase the patterns of people entering and exiting a bus at a specific stop over 24 hours. When a delta occurs a number of spheres are deposited from that stop proportional to the volume of people entering/exiting with colour being utilised to highlight high/low volumes.
⚗ The cumulative effect of spheres being deposited pushes previous ones out to create zones, these zones help identify where clusters of people utilise the network. The concept does get tricky around inner city regions where zones merge but the pattern of occupancy on the periphery of a city is useful for identifying commuter zones/school drops offs etc.
💡 I like this concept as an overview visual, it highlight patterns quickly but in order to be more accurate the spheres would need to be smaller to create distinguishable zones in inner city regions. Or, I guess, 3d pillars could show this data - but it's less fun!
#TransitVizThursday ⚗ Occupancy Heartbeats 🚌
⚡ This week's 3d data visualisation looks at slightly longer-term trends in occupancy and how the heartbeat and pulse of passenger change occurs over a week.
❓ So what is it showing? Similar to last week we are looking at the net change of passenger counts between stops in a rolling 3-hour window. This window gives us enough data to analyse patterns throughout the day. Positive net change ( 🔵) signifies an increase in passenger count between stops and so shows where people are boarding buses and negative net change ( 🔴 ) shows where people are alighting or leaving the bus.
⚗ Each sphere on the map represents a person and has a physicality to it so when multiple people alight the spheres get pushed away creating an effect that looks like growing embers in a fire. The larger zones indicate a greater change in occupancy with the chart at the bottom indicating the total net change in the region for 3-hour bins.
💡 As with last week's experiment this one also has pitfalls, the physicality and weight of the spheres pushing other out to create zones creates a disconnect with the base map. Most of those spheres will be allocated to a few bus stops in the city center so this visual can't be used for accurate insight. What it can give is a decent overview of activity within the region, identifying hotspots or activity outside of the CBD. Plus I find it kind of mesmerising to watch alongside the ambient music!
📊 All data is sourced via BODS (https://lnkd.in/evDazHfU) although this data is taken from an archive a few years ago when passenger counting was more prevalent in the data.
Stay tuned for next weeks #TransitVizThursday - I am always interested in new ideas for how we can analyze transit data; ideas for stories, insight or just experimentation. If you have any I would love to hear your ideas!
#TransitVizThursday: 🗻 Occupancy Mountains in Newcastle 🚍
⚡ I'm trying to keep up the cadence of sharing a mobility-based dataviz every Thursday now - #TransitVizThursday! They won't all be new but worth checking out in case you missed them the first time I posted them.
⚗ This experimental animation takes last week's concept of occupancy change and maps it onto a 3d topographic landscape. When the buses (blue/orange orbs) record a change in occupancy (net change) between stops they deposit balls proportional to this number. These balls form the basis of a landscape that cumulates and grows over the course of 24 hours. The higher the 'mountains' the more people have boarded or alighted the bus over that 24-hour period.
💡 As with any 'experimental' dataviz there are pitfalls, the visual can't be used for detailed insight as the landscape propagation tends to spread along the basemap, however it works as a quick analysis of broad trends across a city. Of course, a simple pillar extrusion would have been simpler and arguably more insightful, but it's not as fun as seeing occupancy mountains form!
#TransitVizThursday: 🚏 Where is your bus filling and emptying? 🚌
A #dataviz exploring the pattern of occupancy data in Newcastle with data via BODS (https://lnkd.in/dz7ym8dr). This is an older project but wanted to re-share it again as I think it's really effective in highlighting the pattern of occupancy data in an area.
I utilise spheres to represent the net change of people on the bus between stops. Blue spheres show people boarding the bus whilst yellow show people alighting. The data only shows us totals so we can only estimate the net change i.e. zero change between stops could mean 2 people board and 2 people alight.
The visualisation also shows two periods of time during the day so morning and afternoon commuter periods can be compared.
Visualising occupancy data is quite complex and creating a slightly abstract depiction of this helps us to comprehend the patterns between space and time within a city centre more clearly